Amazon reveals the three secrets behind the predictive distribution process which underpins its $292-million-dollar success in Australia.
From promises of fast delivery to ultra competitive prices, Amazon’s secret weapon is its use of predictive analytics to power its supply chain, Amazon’s forecasting and capacity lead Jenny Freshwater told the Amazon Web Services Summit in Sydney on Tuesday.
The e-commerce giant’s use of AI and ML to predict demand for product before purchasing and stocking its warehouse is the lynchpin of the retailer’s e-commerce strategy, she says.
“We’re calling this before you click buy because we buy customer obsession in the weeks, days months before you’re on website shopping and decide want to buy something.”
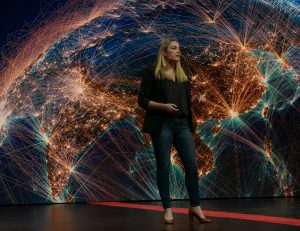
Ms Freshwater said it’s forecast demand which “kicks off the supply chain” and helps Amazon determine which products, and how much of each, to buy.
But the process “is not that simple,” she says, and requires more than just 50 per cent certainty that a product will sell at a point in time.
That’s why the retailer forecasts not just demand for a product but uncertainty around that number, according to Ms Freshwater.
“So when you take hundreds of millions of products and you multiple that by a distribution of potentially 140 data points we’re forecasting every product every day for a whole year. That’s a lot of data,” Ms Freshwater said.
This AI not only has to predict product demand as accurately as possible, but also has to be able to expand rapidly, she says.
“Not only do we have to build and innovate around machine learning and forecast but they have to scale which is potentially the hardest problem we solve.”
Proximity also continues to challenge the retailer, particularly in Australia, where Amazon predicts demand in both cities and in regions.
Three takeaways from Amazon
Amidst the competing challenges of leveraging AI and ML to predict product demand and power the supply chain, Ms Freshwater says there’s three key takeaways the retailer has learnt in the last decade.
- People power
It’s the people that build the algorithms to power AI which are critical to the retailer’s use of ML and AI for its supply chain, she says.
“We have deep learning algorithms that learn from themselves but we still need people, smart scientists to code algorithms. It’s the lifecycle of people that know how to build models that are really key.”
- Data
Investing in good quality data is another crucial lesson learnt by the retailer in their use of AI.
“We spend at least 50 per cent of our development cycle for each model getting the right data. If you put garbage in you’re going to get a bad result,” Ms Freshwater said.
- Collaboration with business
Collaborating with business partners to understand consumer demand is also essential, according to Ms Freshwater.
“We can produce a perfect forecasting model and if it solves the wrong problem or forecasts for the wrong thing its never going to be useful.”